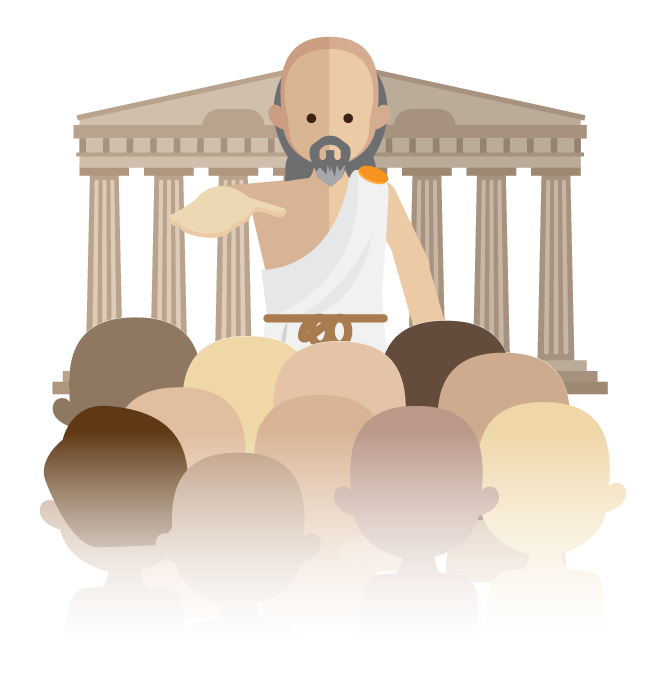 |
|
Why Demand Forecasting is So Important to Supply Chain Professionals and Managers
Hans Levenbach, Executive Director, CPDF Training and Certification Curriculum
|
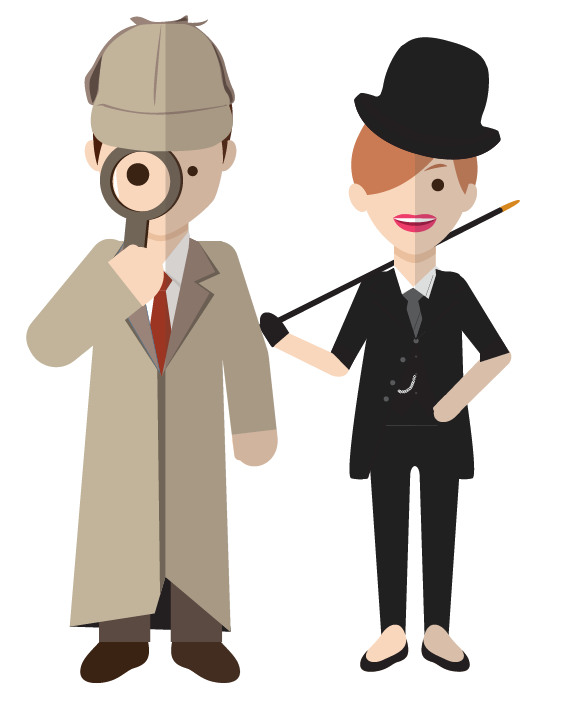 |
|
Smarter Forecasting is Mostly about Data: Improving Data Quality through Data Exploration and Visualization
Hans Levenbach, Executive Director, CPDF Training and Certification Curriculum
|
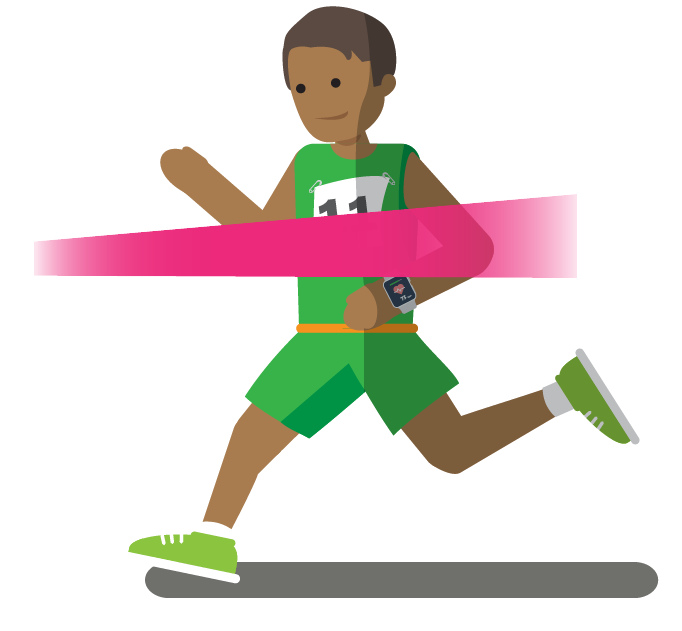 |
|
Predictive Analytics: Selecting Useful Forecasting Techniques
Hans Levenbach, Executive Director, CPDF Training and Certification Curriculum
|
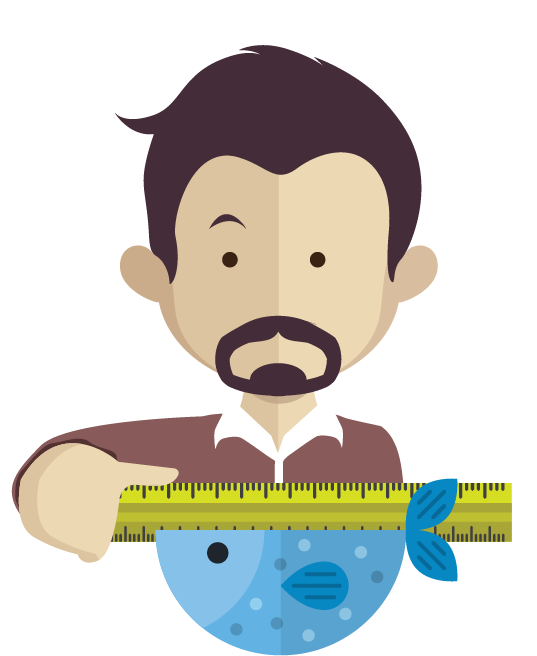 |
|
Taming Uncertainty: What You Need to Know About Measuring Forecast Accuracy
Hans Levenbach, Executive Director, CPDF Training and Certification Curriculum
|
 |
|
The Myth of the Mape - and how to avoid it . . .
Hans Levenbach, Executive Director, CPDF Training and Certification Curriculum
|
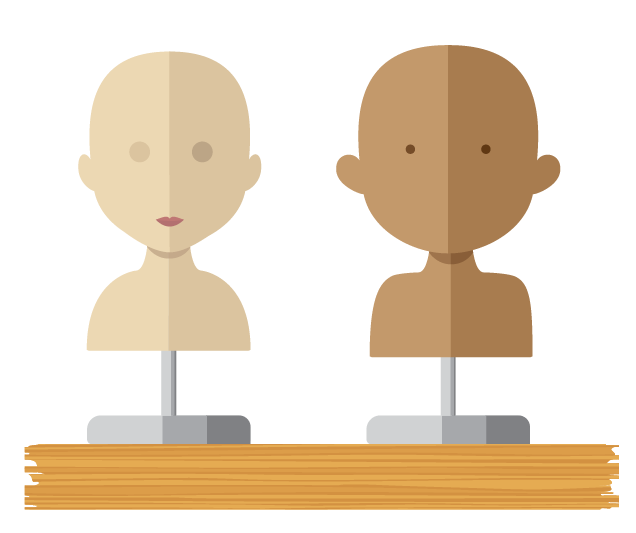 |
|
Characterizing Demand Variability: Seasonality, Trend and the Uncertainty Factor
Hans Levenbach, Executive Director, CPDF Training and Certification Curriculum
|
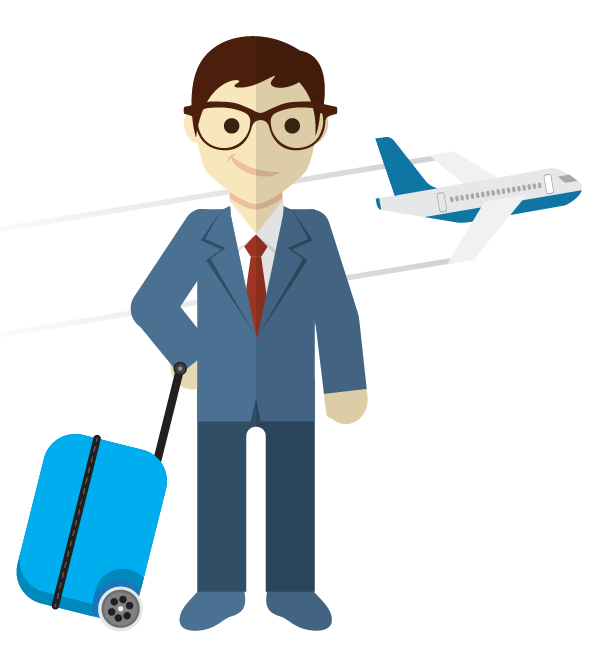 |
|
Dealing With Seasonal Fluctuations
Hans Levenbach, Executive Director, CPDF Training and Certification Curriculum
|
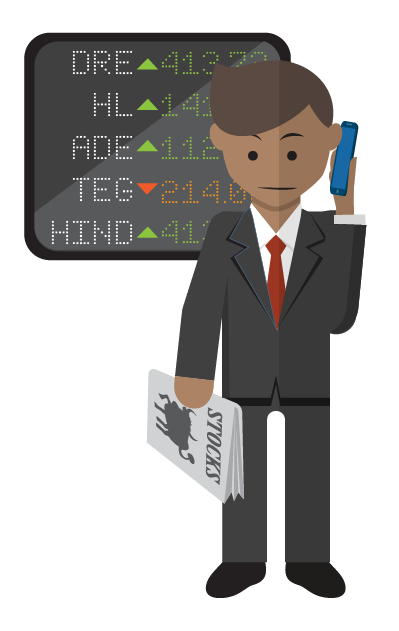 |
|
Trend-Cycle Forecasting with Turning Points
Hans Levenbach, Executive Director, CPDF Training and Certification Curriculum
|
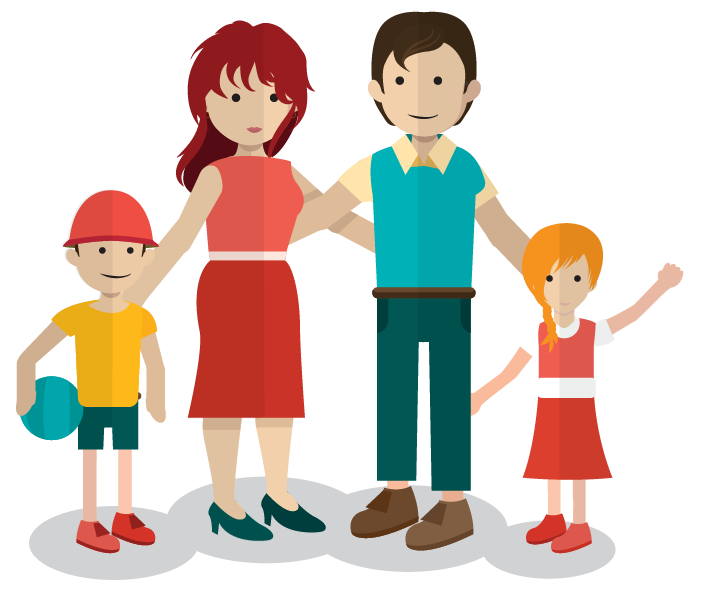 |
|
Big Data: Baseline Forecasting With Exponential Smoothing Models
Hans Levenbach, Executive Director, CPDF Training and Certification Curriculum
|
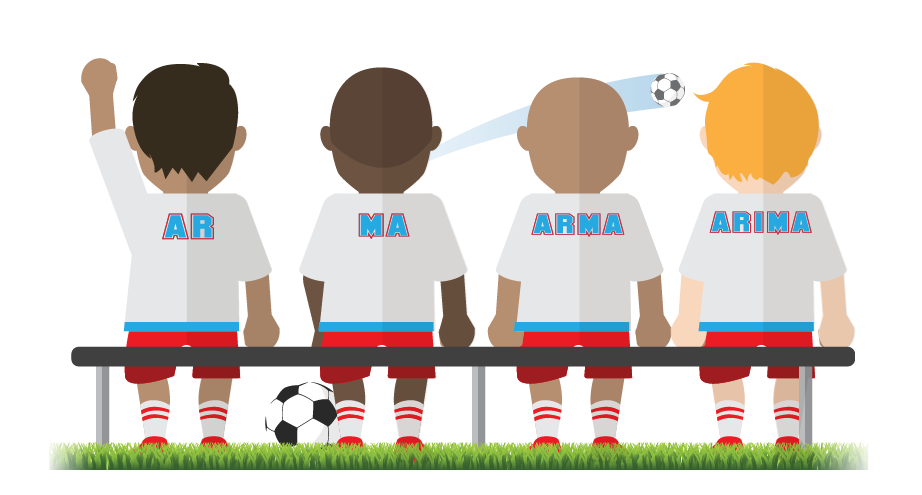 |
|
Short-Term Forecasting with ARIMA Models
Hans Levenbach, Executive Director, CPDF Training and Certification Curriculum
|
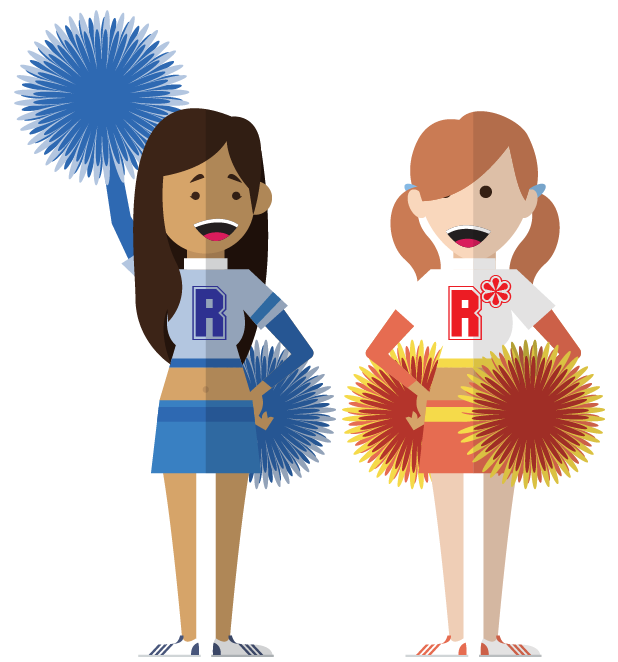 |
|
Demand Forecasting with Regression Models
Hans Levenbach, Executive Director, CPDF Training and Certification Curriculum
|
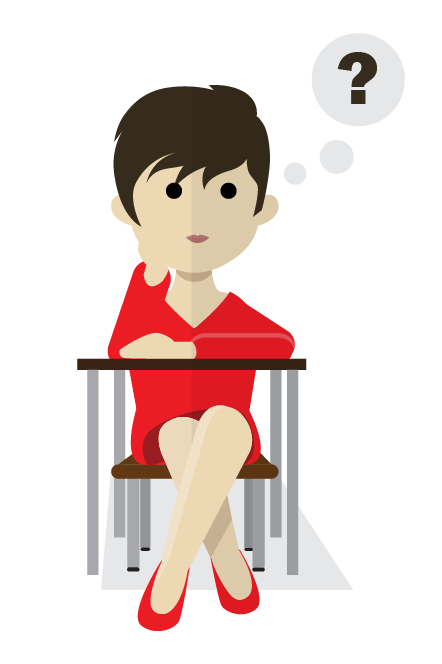 |
|
Gaining Credibility through Root-Cause Analysis and Exception Handling
Hans Levenbach, Executive Director, CPDF Training and Certification Curriculum
|
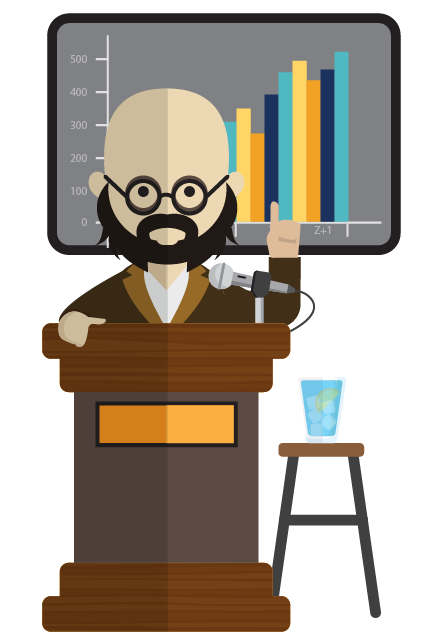 |
|
The Final Forecast Numbers: Reconciling Change and Chance
Hans Levenbach, Executive Director, CPDF Training and Certification Curriculum
|
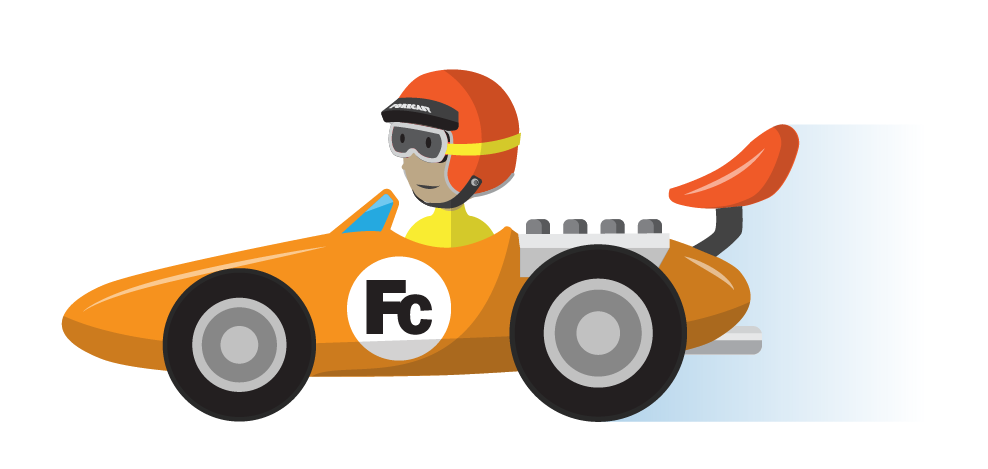 |
|
Creating a Data Framework for Smarter Forecasting and Demand Management
Hans Levenbach, Executive Director, CPDF Training and Certification Curriculum
|
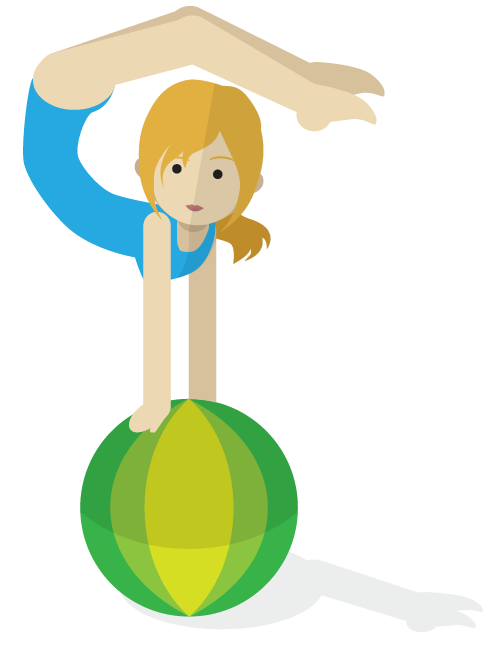 |
|
Blending Agile Forecasting with an Integrated Business Planning Process
Hans Levenbach, Executive Director, CPDF Training and Certification Curriculum
|
 |
|
A Collaborative, Structured Approach to Demand Forecasting in the Supply Chain
Hans Levenbach, Delphus, Inc.
|
 |
|
Measuring Forecaster Performance in a Collaborative Setting with Field Sales Forecasters, Customers or Supplier Partners
Hans Levenbach, Delphus, Inc.
|
 |
|
15-PEERing into the Future: More Accurate and Reliable Forecasts Improve Inventory Planning
Hans Levenbach, Executive Director, CPDF Training and Certification Curriculum
|
 |
|
Predicting the Demand for New Products and Services
Mohsen Hamoudia, International Institute of Forecasters
|
 |
|
Ten Worst (and some Best) Demand Forecasting Practices That Impact Forecasting Performance
Hans Levenbach, Delphus, Inc.
|
 |
|
Demand Management - "Where Practice Meets Theory
Elliott S. Mandelman, CPDF Team Leader
|